Keras入门(四)利用CNN模型轻松破解网站验证码
项目简介
在之前的文章keras入门(三)搭建CNN模型破解网站验证码中,笔者介绍介绍了如何用Keras来搭建CNN模型来破解网站的验证码,其中验证码含有字母和数字。
让我们一起回顾一下keras入门(三)搭建CNN模型破解网站验证码的处理思路:
利用OpenCV对图像进行单个字符的切割,大概400多张图片;
对切割好的单个字符进行人工手动标记;
搭建合适的CNN模型,对标记好的数据集进行训练;
对于新的验证码,先切割单个字符,再对单个字符进行预测,组成总的预测结果。
这一次,笔者将会换种思路,使用CNN模型来破解网站的验证码。我们的数据集如下:
一共是946张图片,这里只展示了一部分,可以看到,这些验证码全部由数字组成。那么,新的破解验证码的思路是什么呢?如下:
- 直接对验证码进行标记,标记的结果见上图;
- 搭建合适的CNN模型对标记好的数据集进行训练;
- 对新验证码进行预测。
这种思路的好处是,不需要对验证码进行繁琐的预处理,只需要简单的数据标记即可。
下面,笔者将会具体展示这个过程。
数据标记
数据标记绝对是个累活,当我想到要对946张图片进行标记并重命名,而且还要保证标记的准确性的时候,我开始是有点拒绝的心态,毕竟这项工作费时费力,而且能不能保证识别的效果还是个未知数。
就这么纠结了一段时间,原本年前就想做的项目一直拖到了现在,后来我想,能不能写个脚本,能够帮助我快速地进行数据标注,并自动保存呢?这么想着,我就动手自己做了一个由Tornado实现的前端页面,可以帮助我快速地标记数据并保存图片,页面如下:
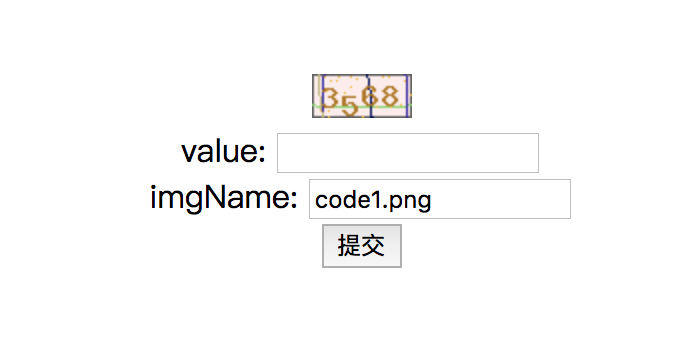
界面虽然简陋,却能帮助我很好地提升数据标记的速度,只需要在value文本框中输入自己识别的结果,程序就能自动保存标记好的图片,并切换至下一张未标记的验证码。有了如此好的工具,结果我用了不到一小时就标记完了这946张验证码(其实是1000张,因为标记好的结果会有重复)。有机会笔者会介绍这个验证码标记的项目~
模型训练
标记完验证码后,我们就利用这946张验证码作为训练数据,训练CNN模型。我们使用Keras框架,CNN模型的结构图如下:
模型训练的Python代码如下:
1 |
|
在这个代码中,我们总共训练了50个epoch,每个epoch共120次批次,每个批次是8张验证码,每张验证码的大小为50*22。
运行该训练模型,后几个epoch的输出结果如下:
总的损失值图像如下:
四个数字每个数字的损失值图像如下:
训练完后,程序会将训练效果最好的epoch保存为best_model.h5文件,便于后续的模型预测。由输出的结果及图像来看,该CNN模型的训练效果应该是相当好的,下面,我们来看看对新验证码的预测效果。
模型预测
新的验证码共有20张,如下:
模型预测的Python代码如下:
1 |
|
运行该模型,得到的输出结果如下:
1 |
|
对这20张新的验证码,预测完全正确!不得不说,CNN模型的识别效果非常好!
总结
本文采用了一种新的思路,搭建CNN模型来实现验证码的识别,取得了不错的识别效果,而且识别的验证码是从网页中下载下来的,具有实际背景,增强了该项目的应用性。
本项目已放至Github,地址为:https://github.com/percent4/CAPTCHA-Recognizition 。
欢迎关注我的公众号NLP奇幻之旅,原创技术文章第一时间推送。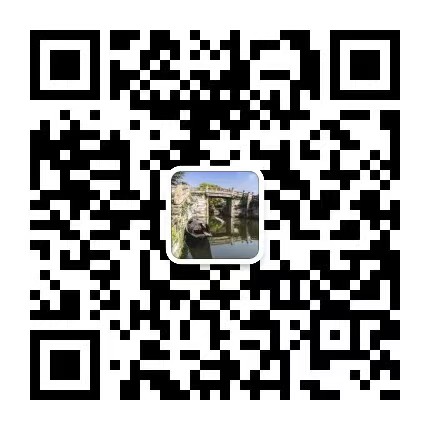
欢迎关注我的知识星球“自然语言处理奇幻之旅”,笔者正在努力构建自己的技术社区。
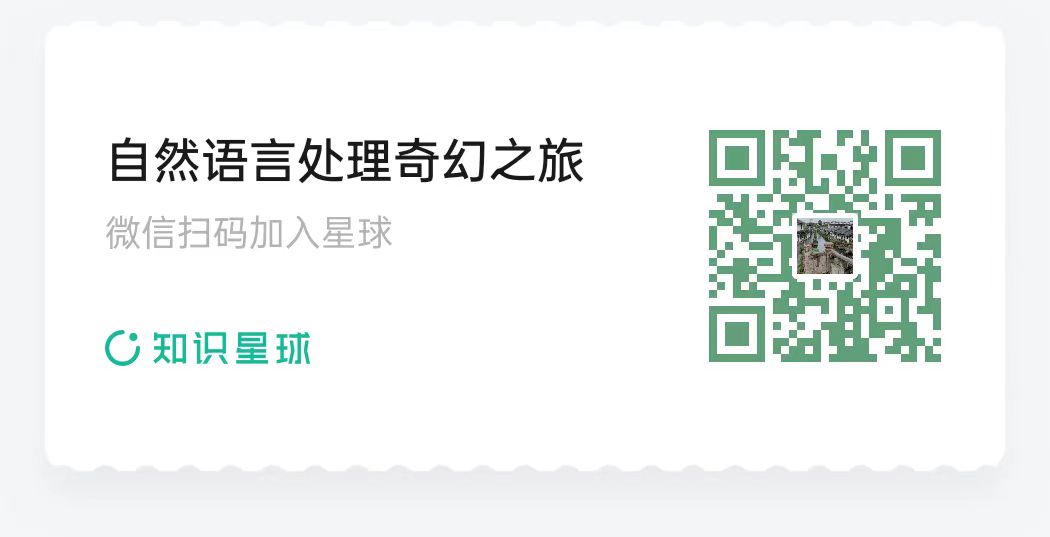