NLP(七十九)函数调用(function_calling)
本文将介绍大模型中的函数调用(function calling),并介绍其在openai, langchain模块中的使用,以及Assistant API对function calling的支持。
函数调用(Function
Calling)是OpenAI
在今年6月13日对外发布的新能力。根据OpenAI官方博客描述,函数调用能力可以让大模型输出一个请求调用函数的消息,其中包含所需调用的函数信息、以及调用函数时所携带的参数信息。这是一种将大模型
(LLM)能力与外部工具/API
连接起来的新方式。
比如用户输入:
What’s the weather like in Shanghai?
使用function
calling,可实现函数执行get_current_weather(location: string)
,从而获取函数输出,即得到对应地理位置的天气情况。这其中,location
这个参数及其取值是借助大模型能力从用户输入中抽取出来的,同时,大模型判断得到调用的函数为get_current_weather
。
开发人员可以使用大模型的function calling能力实现:
- 在进行自然语言交流时,通过调用外部工具回答问题(类似于ChatGPT插件);
- 将自然语言转换为调用API调用,或数据库查询语句;
- 从文本中抽取结构化数据
- 其它
那么,在OpenAI发布的模型中,是如何实现function calling的呢?
本文中,使用的第三方模块信息如下:
1 |
|
入门例子
我们以函数get_weather_info
为例,其实现逻辑(模拟实现世界中的API调用,获取对应城市的天气状况)如下:
1 |
|
该函数只有一个参数:字符串变量city,即城市名称。为了实现function calling功能,需配置函数描述(类似JSON化的API描述),代码如下:
1 |
|
对于一般的用户输入(query),大模型回复结果如下:
1 |
|
此时function_call
为None,即大模型判断不需要function
calling.
对于查询天气的query,大模型输出结果如下:
1 |
|
此时我们看到了令人吃惊的输出,大模型的输出内容为空,而判断需要function
calling,
函数名称为get_weather_info
,参数为{'arguments': '{\n "city": "Beijing"\n}
。
下一步,我们可以调用该函数,传入参数,得到函数输出,并再次调用大模型得到答案回复。
1 |
|
输出结果如下:
1 |
|
以上仅是function calling的简单示例,采用一步一步的详细过程来演示大模型中function calling如何使用。
在实际场景中,我们还需要实现中间过程的函数执行过程。
以下将介绍在OpenAI, LangChain中如何实现function calling。后面我们将使用的3个函数(这些函数仅用于测试,实际场景中可替换为具体的工具或API)如下:
1 |
|
openai调用function calling
在OpenAI的官方模块openai
中实现function calling
的代码如下:
1 |
|
输出结果如下:
1 |
|
LangChain调用function calling
在langchain
中实现function calling
的代码相对简洁写,function calling
的结果在Message中的additional_kwargs变量中,实现代码如下:
1 |
|
OpenAI Assistant API支持function calling
Assistant API
是OpenAI在今年OpenAI开发者大会中提出的创新功能。Assistants API
允许用户在自己的应用程序中构建AI助手。助手有指令,可以利用模型、工具和知识来响应用户查询。Assistants API
目前支持三种类型的工具:代码解释器(Code
Interpreter)、检索(Retrieval)和函数调用(Function
Calling)。
我们来看看,在openai中的Assistant API如何支持function calling。
1 |
|
输出结果如下:
1 |
|
LangChain Assistant API支持function calling
可以看到在openai模块中,在Assistant API中实现function calling,较为麻烦。而新版的langchain(0.0.339)中已经添加对Assistant API的支持,我们来看看在langchain中如何支持function calling。
实现代码如下:
1 |
|
注意,函数get_rectangle_area
为多参数输入,因此需使用StructuredTool.
网页Assistant支持function calling
在OpenAI中的官网中,Assistant已经支持function calling.
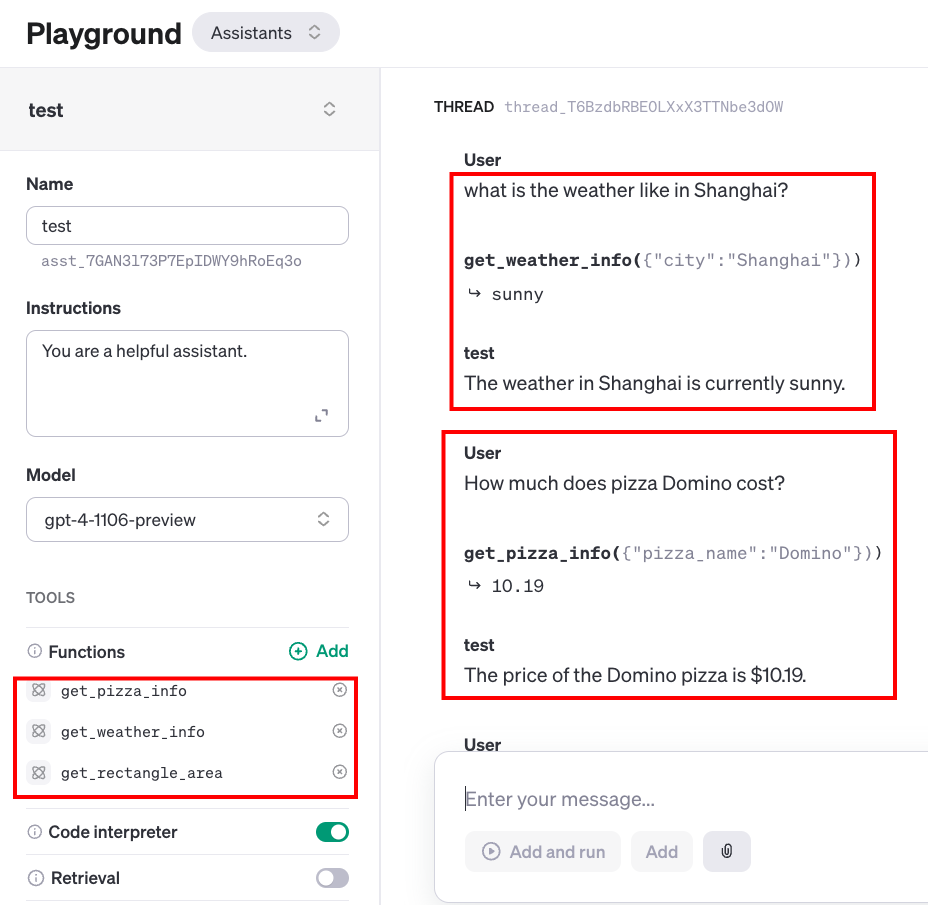
总结
本文是这几天来笔者对于function calling的一个总结。原本以为function calling功能简单好用,但在实际的代码实现中,还是有点难度的,尤其是Assistant API出来后,如何加入外部工具显得尤为重要。
本文作为function calling的一个系统性小结,并给出了详细的代码,希望能对读者有所帮助。
参考文献
- Function calling and other API updates: https://openai.com/blog/function-calling-and-other-api-updates
- OpenAI assistants in LangChain: https://python.langchain.com/docs/modules/agents/agent_types/openai_assistants
- Multi-Input Tools in LangChain: https://python.langchain.com/docs/modules/agents/tools/multi_input_tool
- examples/Assistants_API_overview_python.ipynb: https://github.com/openai/openai-cookbook/blob/main/examples/Assistants_API_overview_python.ipynb
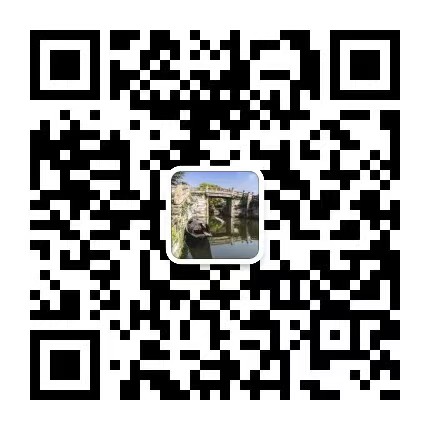
欢迎关注我的知识星球“自然语言处理奇幻之旅”,笔者正在努力构建自己的技术社区。
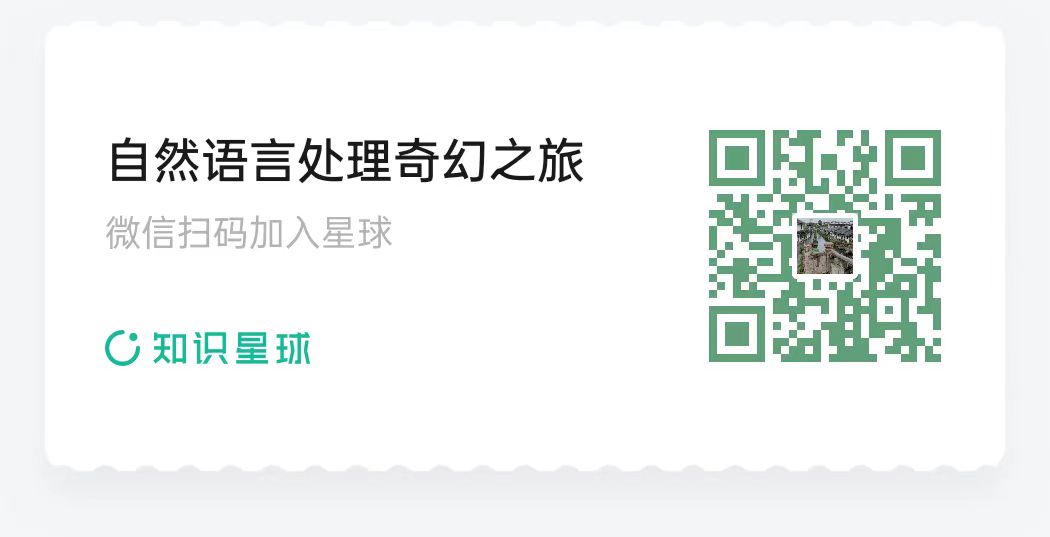