NLP(七十)使用LLAMA-2模型微调Multiple-Choice-MRC
本文将介绍如何在Firefly大模型训练框架中,使用LLAMA-2 7B模型,对多项选择阅读理解数据集RACE middle进行微调,最终效果提升明显。
MRC
机器阅读理解(Machine Reading Comprehension, MRC)
属于NLP任务中的问答任务(Question
Answering,
QA),是NLP领域中基础且重要的一项任务。所谓的机器阅读理解就是给定一篇文章,以及基于文章的一个问题,让机器在阅读文章后对问题进行作答。
根据任务形式的不同,可划分为:
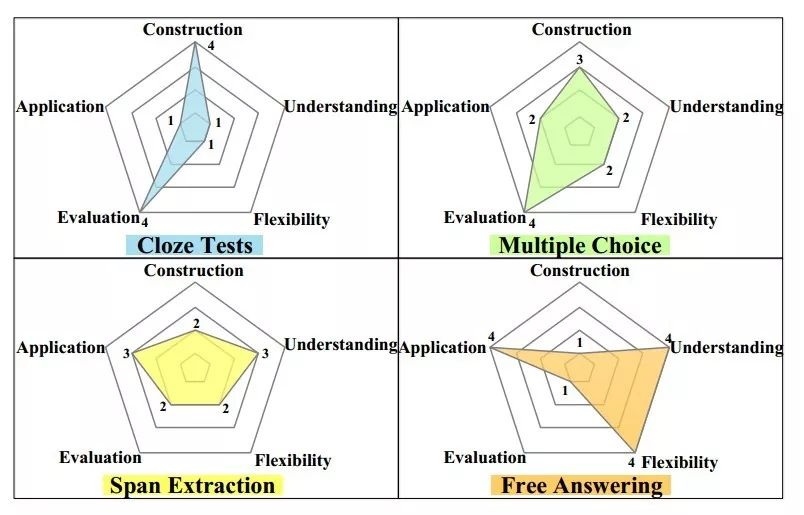
完形填空(Cloze Tests):将文章中的某些单词隐去,让模型根据上下文判断被隐去的单词最可能是哪个。
多项选择(Multiple Choice):给定一篇文章和一个问题,让模型从多个备选答案中选择一个最有可能是正确答案的选项。
片段抽取(Span Extraction):给定一篇文章和一个问题,让模型从文章中抽取连续的单词序列,并使得该序列尽可能的作为该问题的答案。
自由作答(Free Answering):给定一篇文章和一个问题,让模型生成一个单词序列,并使得该序列尽可能的作为该问题的答案。与片段抽取任务不同的是,该序列不再限制于是文章中的句子。
其中,完形填空
是BERT模型中的其中一项预训练任务MLM,因此,BERT系列的模型天然支持完形填空。
片段抽取
即抽取式阅读理解,是我们在NLP任务中最常见的阅读理解的形式,一般情况下的阅读理解也指的是这种形式。使用BERT系列模型也能很好地完成该任务。该任务的经典数据集有SQUAD, SQUAD 2等。
自由作答
不限定回复的答案来自于原始文章,因此难度最大,一般采用生成式模型解决,以前的NLP模型在这方面的表现不佳。而大模型(LLM)的出现,让该任务有了很大改观,效果有了较强的阅读性,属于革命性的变化。
多项选择
即我们在英语考试中的阅读理解,阅读文章,给定问题,从固定的选项中选出正确答案。经典的多项选择阅读理解数据集有RACE , SWAG等。本文将会具体介绍多项选择阅读理解中的RACE数据集,以及LLAMA-2
在该数据集上的微调效果。
RACE数据集
RACE
数据集是多项选择阅读理解(Multi Choice
MRC)中的经典数据集,RACE官方网址
中的介绍为:
Race is a large-scale reading comprehension dataset with more than 28,000 passages and nearly 100,000 questions. The dataset is collected from English examinations in China, which are designed for middle school and high school students. The dataset can be served as the training and test sets for machine comprehension.
RACE数据集又分为middle(初中)和high(高中)两部分,如下:
数据集 | train | dev | test |
---|---|---|---|
middle | 25421 | 1436 | 1436 |
high | 62445 | 3451 | 3498 |
total | 87866 | 4887 | 4934 |
由于数据规模原因和训练时间原因,本文只对middle数据集进行微调。随机从middle数据集中的训练集挑选一条样本,如下:
1 |
|
构建Prompt
本文针对RACE数据集,构建的Prompt如下:
Read the following passage and questions, then choose the right answer from options, the answer should be one of A, B, C, D.
<passage>:
"I planted a seed. Finally grow fruits. Today is a great day. Pick off the star for you. Pick off the moon for you. Let it rise for you every day. Become candles burning myself. Just light you up, hey!... You are my little little apple. How much I love you, still no enough."
This words are from the popular song You Are My Little Dear Apple. Bae Seul-Ki acted as the leading dancer in the MV of the song. She loves dancing. She became crazy about hip-hop when she was a school girl.
Bai Seul-Ki was born on September 27, 1986. She is a South Korean singer and dancer. She is 168cm tall. She loves cooking. Her favourite food is spicy and salty. She like pink and red most. There are five members in her family---father, mother, two younger brothers and herself. She isn't married.
After her father and mother broke up, she lived with her mother and new daddy. She enjoys being alone.
<question>:
Bae Seul-Ki _ in the MV of the song according to the passage.
<options>:
A sang
B danced
C cried
D laughed
<answer>:
Trick: 可以借助大模型,比如GPT-4构建能好的Prompt以提升微调效果。
LLAMA-2模型微调
在大模型领域,LLAMA
系列模型可谓大名鼎鼎,其本身及衍生的模型有几十个,真让人眼花缭乱,而且效果都很不错,是大模型领域真正的一代宗师。我们有机会再介绍LLAMA系列模型,本文不再详述。
本文使用刚开源的LLAMA 2
模型,7B版本,在Firefly
框架下,对RACE
middle数据集进行微调。微调的参数如下:
1 |
|
在文章NLP(六十三)使用Baichuan-7b模型微调人物关系分类任务,我们已经详细介绍了Firefly模型微调、评估、WEB服务等步骤,这里也不再详述。也可以参考Github项目llama-2-multiple-choice-mrc .
在RACE test数据集上的准确率为86.91%
!效果可谓惊人,而这只是LLAMA
2没有经过参数调整的结果,效果不可谓不佳。笔者之前用BERT模型同样在RACE
middle数据集上进行微调,评估结果一般只有72%左右,BERT
Large模型才不过75%,这还是训练数据采用了middle + high的结果。
RACE数据集排行榜如下:
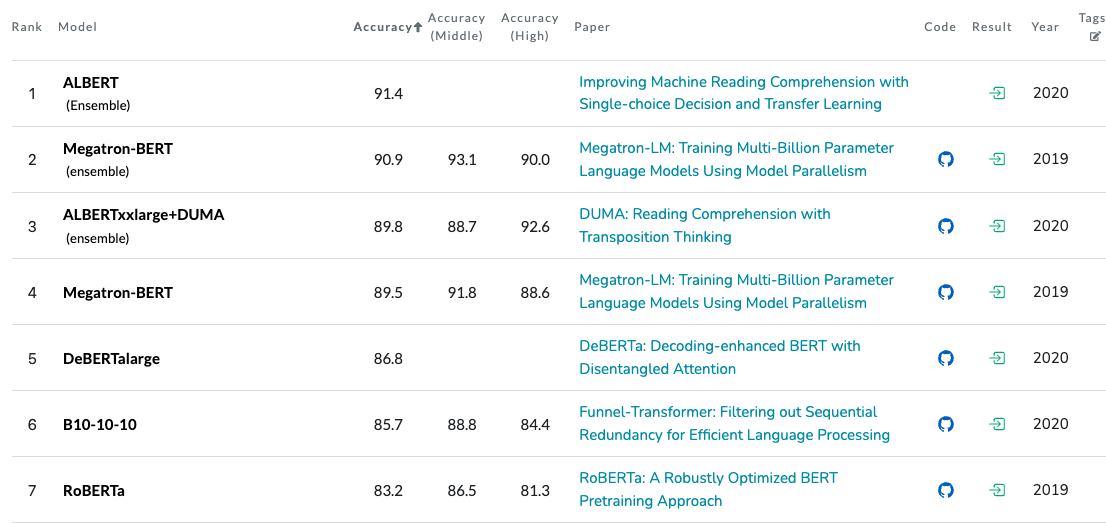
模型效果评估
最后,我们在新的文章上进行评估。我们从网上随机选取一篇初中英语阅读理解文章,如下:
Edward rose early on the New-year morning. He looked in every room and wished a Happy New Year to his family. Then he ran into the street to repeat that to those he might meet.
When he came back, his father gave him two bright, new silver dollars.
His face lighted up as he took them. He had wished for a long time to buy some pretty books that he had seen at the bookstore.
He left the house with a light heart, expecting to buy the books. As he ran down the street, he saw a poor family.
“I wish you a Happy New Year.” said Edward, as he was passing on. The man shook his head.
“You are not from this country.” said Edward. The man again shook his head, for he could not understand or speak his language. But he pointed to his mouth and to the children shaking with cold, as if (好像) to say, “These little ones have had nothing to eat for a long time.”
Edward quickly understood that these poor people were in trouble. He took out his dollars and gave one to the man, and the other to his wife.
They were excited and said something in their language, which doubtless meant, “We thank you so much that we will remember you all the time.”
When Edward came home, his father asked what books he had bought. He hung his head a moment, but quickly looked up.
“I have bought no books”, said he. “I gave my money to some poor people, who seemed to be very hungry then.” He went on, “I think I can wait for my books till next New Year.”
“My dear boy,” said his father, “here are some books for you, more as a prize for your goodness of heart than as a New-year gift”
“I saw you give the money cheerfully to the poor German family. It was nice for a little boy to do so. Be always ready to help others and every year of your life will be to you a Happy New Year.”
四个问题如下:
48. Edward expected to _________ with the money he got from his father.
A. help the poor family B. buy something to eat
C. buy some pretty books D. learn another language
49. Why did the poor man shake his head when Edward spoke to him?
A. He couldn’t understand the boy B. He wouldn’t accept the money
C. He didn’t like the boy’s language D. He was too cold to say anything
50. How much did Edward give the poor family?
A. One dollar B. Two dollars C. Three dollars D. Four dollars
51. We know that Edward_________ from the passage?
A. got a prize for his kind heart B. had to buy his books next year
C. bought the books at the bookstore D. got more money from his father
微调模型给出的答案为CABA,与参考答案一致!
总结
多项选择阅读理解一直是笔者这几年来关注的人物,而之前的BERT时代,BERT模型的效果并不太好,Megatron-BERT将指标提升至90%左右,这中间付出了太多努力,效果也不太好复现。笔者一直都在做这方面的努力,而囿于机器资源或模型参数,都未能成功。LLAMA系列模型的出现,让这一切变得如此轻松写意,虽然效果还不是SOTA,但效果无疑是让人满意的。大模型无疑是未来人工智能的潮流,是当今时代的弄潮儿!
本文主要介绍了MRC及RACE数据集,同时介绍了如何在Firefly训练框架下,采用LLAMA 2模型进行微调,并取得了满意的效果。
欢迎关注我的公众号NLP奇幻之旅,原创技术文章第一时间推送。
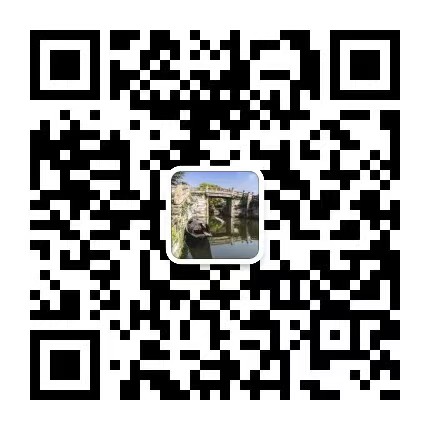
欢迎关注我的知识星球“自然语言处理奇幻之旅”,笔者正在努力构建自己的技术社区。
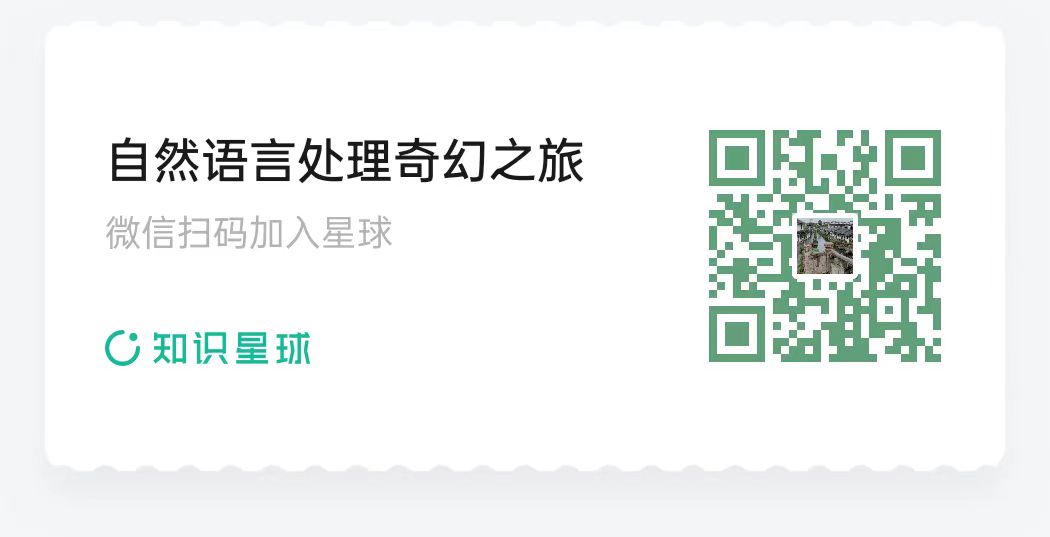