NLP(二十八)多标签文本分类
本文将会讲述如何实现多标签文本分类。
什么是多标签分类?
在分类问题中,我们已经接触过二分类和多分类问题了。所谓二(多)分类问题,指的是y值一共有两(多)个类别,每个样本的y值只能属于其中的一个类别。对于多标签问题而言,每个样本的y值可能不仅仅属于一个类别。
举个简单的例子,我们平时在给新闻贴标签的时候,就有可能把一篇文章分为经济和文化两个类别。因此,多标签问题在我们的日常生活中也是很常见的。
对于多标签问题,业界还没有很成熟的解决方法,主要是因为标签之间可能会存在复杂的依赖关系,这种依赖关系现阶段还没有成熟的模型来解决。我们在解决多标签问题的时候,一种办法是认为标签之间互相独立,然后把该问题转化为我们熟悉的二(多)分类问题。
本文以 2020语言与智能技术竞赛:事件抽取任务 中的数据作为多分类标签的样例数据,借助多标签分类模型来解决。
整个项目的结构如下图所示:
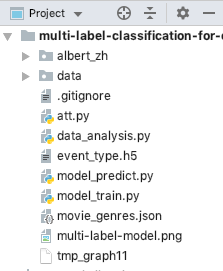
首先,让我们来看一下样例数据。
数据分析
首先,让我们来看一下样例数据的几个例子:
司法行为-起诉|组织关系-裁员 最近,一位前便利蜂员工就因公司违规裁员,将便利蜂所在的公司虫极科技(北京)有限公司告上法庭。 组织关系-裁员 思科上海大规模裁员人均可获赔100万官方澄清事实 组织关系-裁员 日本巨头面临危机,已裁员1000多人,苹果也救不了它! 组织关系-裁员|组织关系-解散 在硅谷镀金失败的造车新势力们:蔚来裁员、奇点被偷窃、拜腾解散
从上面的例子中我们可以看出,同样的描述文本,有可能会属于多个事件类型。比如上面的在硅谷镀金失败的造车新势力们:蔚来裁员、奇点被偷窃、拜腾解散
,该句话中包含了组织关系-裁员
和组织关系-解散
两个事件类型。
该数据集中的训练集一共有11958个样本,65个事件类型,我们对该训练集进行简单的数据分析,来看看多事件类型的个数和占比,以及每个事件类型的数量。数据分析的脚本如下:
1 |
|
输出结果如下:
1 |
|
模型训练
我们利用sklearn模块中的MultiLabelBinarizer进行多标签编码,如果文本所对应的事件类型存在,则将该位置的元素置为1,否则为0。因此,y值为65维的向量,其中1个或多个为1,是该文本(x值)对应一个或多个事件类型。
我们采用ALBERT对文本进行特征提取,最大文本长度为200,采用的深度学习模型如下:
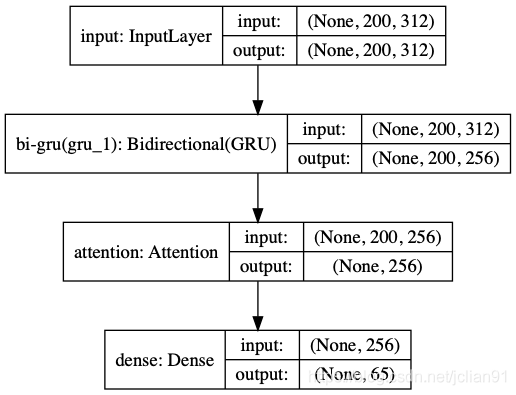
模型训练的脚本(model_trian.py)的代码如下:
1 |
|
训练过程输出内容如下:
1 |
|
在最终的epoch上,训练集上的acuuracy为0.9966,测试集上的acuuracy为0.9964。训练结果的loss和acc曲线如下:
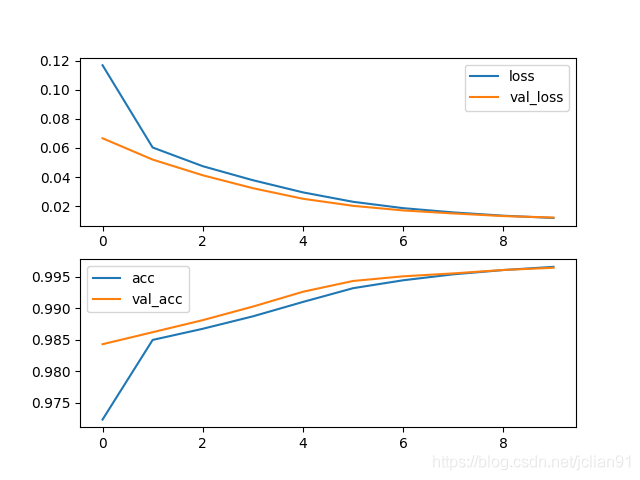
从上述结果看,多标签分类的模型效果还是相当不错的。
模型预测
我们利用下面的模型预测脚本(model_predict.py)对新的测试集数据进行验证,脚本代码如下:
1 |
|
其中的几个样本的预测结果如下:
预测语句: 北京时间6月7日,中国男足在广州天河体育场与菲律宾进行了一场热身赛,最终国足以2-0击败了对手,里皮也赢得了再度执教国足后的首场比赛胜利! 预测事件类型: 竞赛行为-胜负
预测语句: 巴西亚马孙雨林大火持续多日,引发全球关注。 预测事件类型: 灾害/意外-起火
预测语句: 19里加大师赛资格赛前两天战报 中国选手8人晋级6人遭淘汰2人弃赛 预测事件类型: 竞赛行为-晋级
预测语句: 日本电车卡车相撞,车头部分脱轨并倾斜,现场起火浓烟滚滚 预测事件类型: 灾害/意外-车祸
预测语句: 截止到11日13:30 ,因台风致浙江32人死亡,16人失联。具体如下:永嘉县岩坦镇山早村23死9失联,乐清6死,临安区岛石镇银坑村3死4失联,临海市东塍镇王加山村3失联。 预测事件类型: 人生-失联|人生-死亡
预测语句: 定位B端应用,BeBop发布Quest专属版柔性VR手套 预测事件类型: 产品行为-发布
预测语句: 8月17日。凌晨3点20分左右,济南消防支队领秀城中队接到指挥中心调度命令,济南市中区中海环宇城往南方向发生车祸,有人员被困。 预测事件类型: 灾害/意外-车祸
预测语句: 注意!济南可能有雷电事故|英才学院14.9亿被收购|八里桥蔬菜市场今日拆除,未来将建新的商业综合体 预测事件类型: 财经/交易-出售/收购
预测语句: 昨天18:30,陕西宁强县胡家坝镇向家沟村三组发生山体坍塌,5人被埋。当晚,3人被救出,其中1人在医院抢救无效死亡,2人在送医途中死亡。今天凌晨,另外2人被发现,已无生命迹象。 预测事件类型: 人生-死亡|灾害/意外-坍/垮塌
总结
本项目已经上传至Github项目,网址为:https://github.com/percent4/multi-label-classification-4-event-type 。
后续有机会再给大家介绍更多多标签分类相关的问题,欢迎大家关注~
欢迎关注我的公众号NLP奇幻之旅,原创技术文章第一时间推送。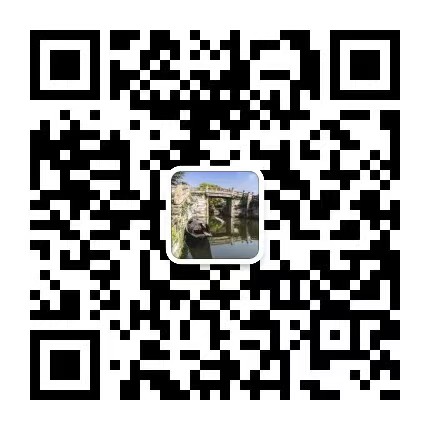
欢迎关注我的知识星球“自然语言处理奇幻之旅”,笔者正在努力构建自己的技术社区。
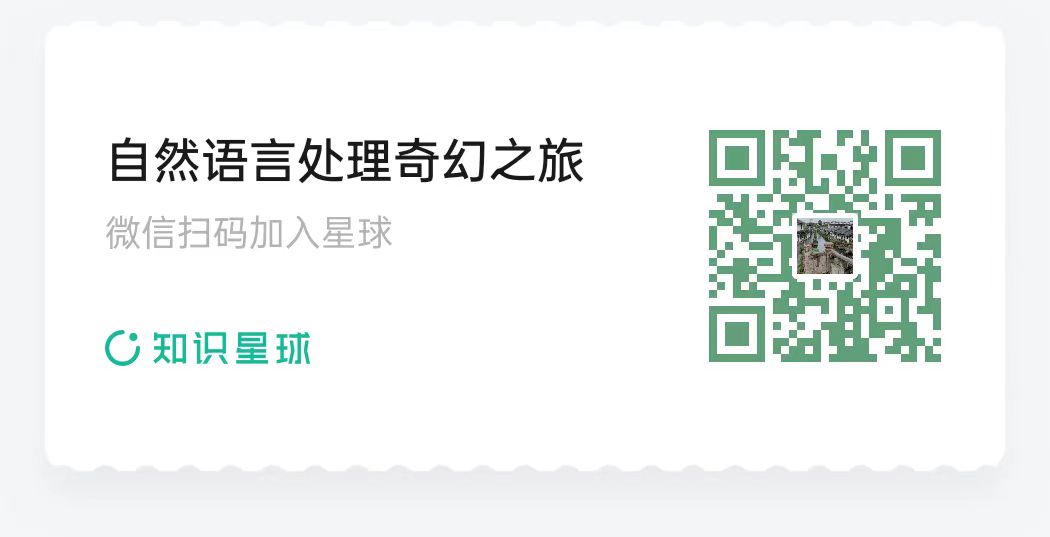